How PI.EXCHANGE’s AI & Analytics Engine helped an inter-modal maritime logistics provider shift to an anticipatory strategy based on accurate demand forecasting to achieve greater operational efficiency
The logistics industry has access to an increasing volume of data, their challenge is that the conventional methods to extract business intelligence have required large investments in internal resources, expertise, and infrastructure in order to synthesize all of the data and enable data-driven decision-making.
The Challenge: Predict the itinerary of competitor vessels
Working with an Inter-modal maritime logistics company, PI.EXCHANGE Platform presented a resource-efficient solution to extract business intelligence from data. The company sought to predict the itinerary of their competitors' vessels, to better understand the short-term fluctuations in global shipment volumes. However, their current processes, reflective of the industry at large were labor-intensive and required specialized staff and domain knowledge. PI.EXCHANGE’s AI & Analytics Engine was able to efficiently assist the company in forecast demand, forecast demand provided actionable insight to:
- Understand extra capacity needed in order to meet unexpected demand;
- Reduce kilometers spent re-positioning assets;
- Increase cargo capacity utilization; and
- Increase asset utilization for asset owners.
Improvements via the AI & Analytics Engine in short-range forecasting demand included an increase in the accuracy of competitors' port itinerary prediction from 20% to 90% and significant savings in both time and resources normally required to extract business intelligence from data. Subsequently, the company increased operational efficiency for less expenditure. For logistics providers, profit margins can rise when those unnecessary costs are eliminated and demand forecasting is accurate, and in a highly competitive market, this provided a significant competitive edge.
For other relevant use cases, check out our article on the Top 18 essential AI Use Cases in Leading Industries!
Industry Challenge:
As an increasing population demand for goods increases, the logistics industry is under pressure to enhance efficiencies and effectiveness in the delivery of logistical services.
However, the rather traditional maritime logistics industry has been slow to evolve and innovate, presenting an opportunity for providers willing to do so, to build a competitive edge. Logistics companies at present generate a large amount of data internally and have access to even more publicly available. Though, the challenge remains that the internal resources, expertise and infrastructure to synthesize all of this information and enable data-driven decision-making continues to represent a significant barrier.
With the help of predictive optimization, logistics companies can shift to an anticipatory strategy based on accurate demand forecasting, and thereby achieve far greater operational efficiency in a slow to innovate but highly competitive industry.
PI.EXCHANGE provides a solution to the conventional methods and its inherent problems through a resource efficient AI & Analytics Engine, ultimate removing the barriers to accessing business intelligence.
Maritime transport is arguably the single leading logistic operation in the transportation of goods. The global value of goods transported according to HSBC’s Trade Flow Report (2017) is expected to develop to a value of US$68.5 trillion by 2050.
Those that can gain a competitive edge through their data stand to represent a larger slice of this highly competitive market.
How the AI & Analytics Engine provided actionable business intelligence
Data Wrangling
Through the smart data wrangling feature the itinerary of each of the vessels from their attributes was computed. The itinerary is made up of:
- The next four ports visits by the vessel from the time of observation;
- And contains the time spent at each port.
Model Recommendation & Selection
Machine learning was subsequently used to construct models to best predict the upcoming itinerary of each vessel. By recommending the model with AI, significant time was saved not having to brute force an optimal model fit for the data. The Model Recommender ranks our expertly-crafted model templates by their Predictive Performance, Training Time, and Prediction Time metrics, models can then be tested for performance.
Deployment
With a few clicks of a button the best model can be deployed. The top two predictions from the respective models predicted the next port itinerary with 90% accuracy and days at port with 85% accuracy.
Prediction Outputs
Once deployed, the model's corresponding API endpoint is available immediately for use. To increase access to predictions for the Client, predictions are routed to a web-app that calls the prediction endpoint.
End-to-end life-cycle management
PI.EXCHANGE Platform catalogues all models created and of the models deployed automatically and continuously monitors performance. Due to fast-moving nature of the shipping business this feature is highly valued so the team can consistently make smarter faster business decisions. If performance deteriorates, PI.EXCHANGE is able to train new models within minutes.
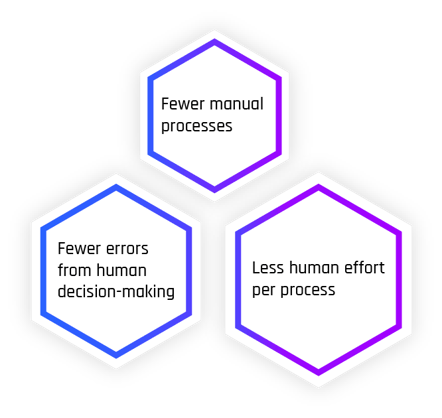
Results & Benefits
Without the AI & Analytics Engine, the process to determine the itinerary of vessels was labor intensive and required individuals with significant experience and domain knowledge sifting through large and often un-sanitized datasets.
Improvements via the AI & Analytics Engine included an increase in the accuracy of prediction from 20% to 90%, a reduction of internal processing from 5+ days to less than 1 day and, an automatic and continuous view of model health, this assisted in:
- Optimizing operations to significantly reduce costs;
- Increase in employee efficiency;
- Optimal Fleet re-positioning; and
- Selling extra logistics assets.